Welcome to Alps Agility
Your Expert in Crafting Data Platforms, Cloud Transitions, and Optimization.
Alps Agility is a data consultancy that specializes in building data platforms, migrating to the cloud, and optimizing data platforms on-prem and Cloud. We are a certified Google Cloud Partner and EDMCouncil Partner, and we have a team of experienced and certified data engineers and architects who are passionate about helping businesses get the most out of their data through building scalable data infrastructure for the future.
Empower businesses by assisting them in building and optimising their data platforms
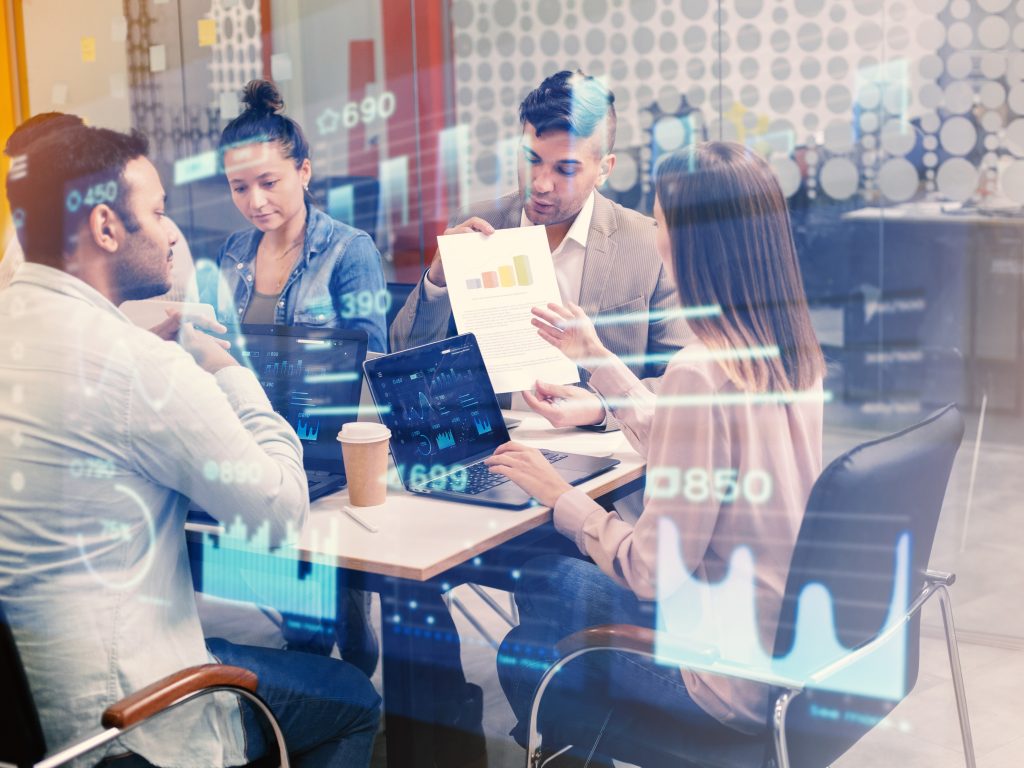
We firmly believe that data is the linchpin of success in today’s dynamic digital landscape. Our mission is to empower businesses by assisting them in building and optimising their data platforms, whether on-premises or in the Cloud. While we offer solutions that cater to both on-premises and Cloud environments, the real transformative benefits can be witnessed most distinctly when we collaborate in a cloud-based setting. Our founder has extensive experience in working with open-source Hadoop technologies and has actively contributed to the open-source community.
Empower our client partners to make more informed decisions, enhance the efficiency of their operations
By harnessing our expertise, we empower our client partners to make more informed decisions, enhance the efficiency of their operations, and ultimately drive substantial business growth. We understand that every business has unique requirements, and we tailor our solutions to meet those specific needs. Our main aim is to be the trusted partner for businesses of all sizes in managing their data. With our unwavering commitment to excellence, we ensure our clients receive top-quality services and continuous support on their data journey, whether it’s on-site, in the Cloud, or with open-source technologies.